

However, cunningly chosen parsimonious models often do provide remarkably useful approximations.” As Box (1979) says, “t would be very remarkable if any system existing in the real world could be exactly represented by any simple model. This fact was nicely captured in the title of Theodore Micceri’s (1989) paper, “The Unicorn, the Normal Curve, and Other Improbable Creatures.” The normal curve does not occur in nature, but it’s a very useful mathematical model – rather like the points and lines of elementary geometry. No model can capture all of the niceties of the real world, so that (theory-driven) models are idealizations and simplifications. He gave an example: “In many circumstances even though no theoretical model is available, perfectly good empirical approximations can be obtained by fitting a polynomial or some other flexible graduating function over the region of interest” (2005).īox’s point that models are approximations applies to theory-driven models as well as empirical models. Theory-driven models, on the other hand, can be wrong, inadequate, or indeed wildly misleading descriptions of the reality they are being used to represent. Data-driven models cannot be wrong–though they can be poor, or of varying degrees of usefulness for any particular purpose–because they are simply summarizing data and are not describing any purported underlying reality. In the context of the two model types, we see that this comment is not quite right. The distinction between the two types of model comes into focus when we recall an even more famous comment than Anderson’s, which is George Box’s remark to the effect that, while all models are wrong, some models are useful (e.g. Certainly, a given statistical technique might be used to fit models of either type. Moreover, models often start out as data-driven and gradually become theory-driven as understanding grows. The two types of model need not be exclusive–both can be used in any particular application, and indeed, the division between the two types may not always be sharp. In contrast, data-driven models merely seek to summarize or describe the data. Theory-driven models encapsulate some kind of understanding (theory, hypothesis, conjecture) about the mechanism underlying the data, such as Newton’s Laws of motion in mechanics, or prospect theory in psychology. On the one hand we have theory-driven, theoretical, mechanistic, or iconic models, and on the other hand we have data-driven, empirical, or interpolatory models. However, for data science I think the key distinction-at least as far as responding to Anderson’s comment goes-lies between two types of model, which appear under various names. Cox 1990 McCullagh 2002 Neyman and Scott 1959), and computer scientists use the term “data model” to describe the relationships between the aspects of the structure of a data set. Some authors add other types of model, or make other distinctions (e.g. He failed to take account of the fact that there are two fundamentally different types of model, and that while “big data” might partly replace one, it will not do so for the other. This notion that having enough data means we do not have to worry about constructing models invokes the saying that “the numbers speak for themselves,” although this adage has something of a history (see Hand 2019).īut Anderson was wrong. If we can instead simply crunch vast data sets, relying on the awesome power of modern computers, so much the better. After all, coming up with realistic models describing the way (natural) processes might work is hard mental effort. The notion that we can manage without models and that sufficient quantities of data-big data-can take the place of models is a seductive one.

We can throw the numbers into the biggest computing clusters the world has ever seen and let statistical algorithms find patterns where science cannot.” As an example, he cites Craig Venter’s sequencing of genotypes. We can analyze the data without hypotheses about what it might show. Indeed, they don't have to settle for models at all.” He went on to say, “We can stop looking for models.
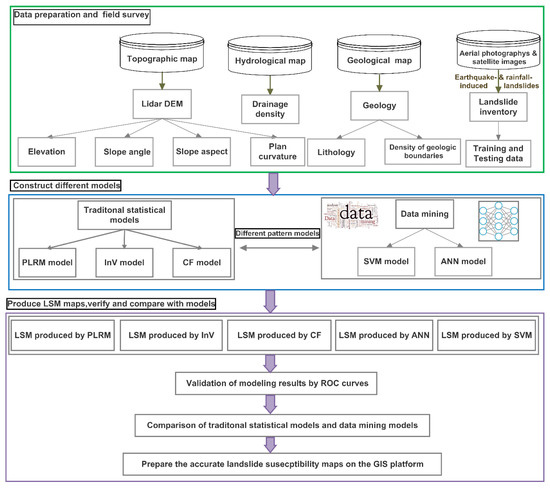
Chris Anderson, the former editor of Wired magazine, famously wrote (2008) that “oday companies like Google, which have grown up in an era of massively abundant data, don't have to settle for wrong models.
